EVENT
ARTIFICIAL INTELLIGENCE IN WEALTH MANAGEMENT
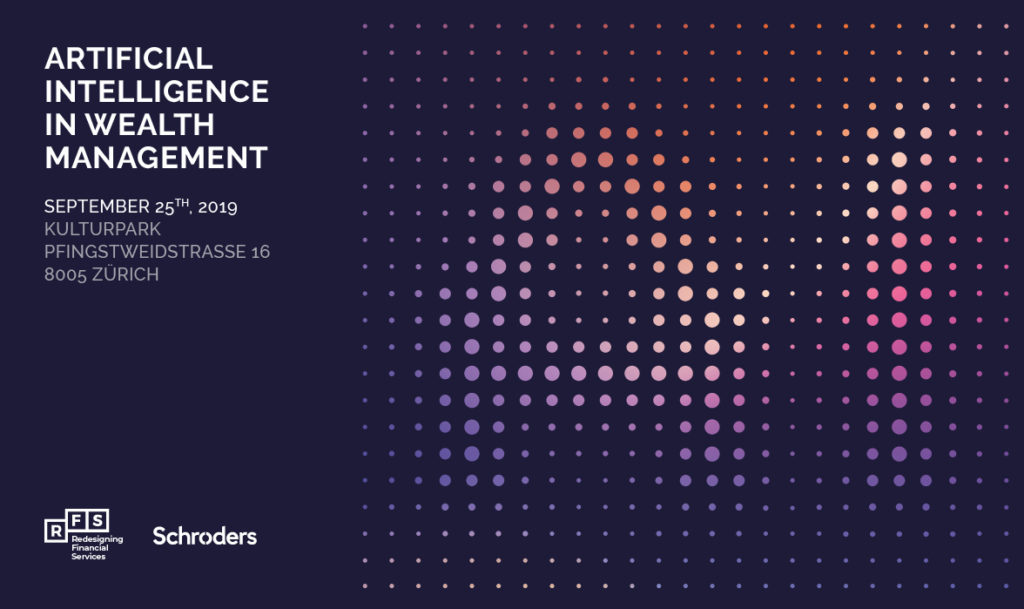
Artificial Intelligence’s benefits are manifold and there is barely an industry that won’t be affected by automation in the coming years. The financial services industry, often at the forefront of technology adoption, offers an especially interesting opportunity for the deployment of AI technologies.
In recent years, however, the term has been used exponentially. Distinguishing hype from reality isn’t that easy anymore.
Cutting through the noise: What are the real value-adding applications and benefits of AI in Wealth Management?
The event sheds light on the challenges in the Wealth Management industry, the potential of Artificial Intelligence as well as different perspectives on real-world use cases of AI in Wealth Management.
Schedule
18:00 – 18:15
Introduction
by Zani Sharifi, RFS
18:15 – 18:30
Challenges in Wealth Management
by Olaf Toepfer, EY
18:30 – 18:40
Empowering investment selection with machine learning
by David Machac, Schroders Adveq
18:40 – 18:50
The Wealth of AI Management
by Henrique Saeuberli, IBM Research ThinkLab
18:50 – 19:00
Fueling every stage of the investment process with AI generated analytics
by Anders Bally, Sentifi
19:00 – 19:10
AI-driven Wealh Management
by Dorian Selz, Squirro
19:10 – 19:20
The cloud is driving Artificial Intelligence
by Martin Moeller, Microsoft
19:20 – 20:00
Panel (with Olaf Toepfer, Henrique Saeuberli, Dorian Selz and Martin Moeller)
Speakers
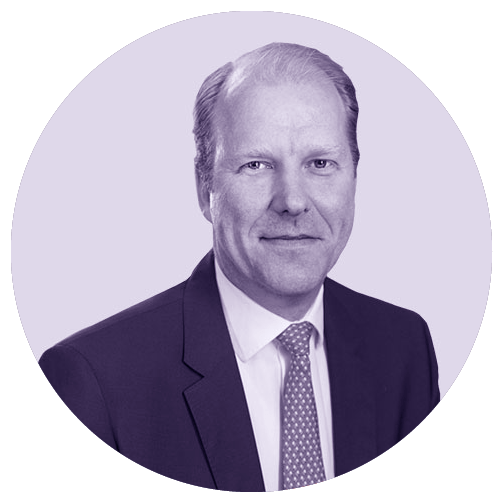
Olaf Toepfer
Senior Partner and Head of Banking & Capital Markets, EY
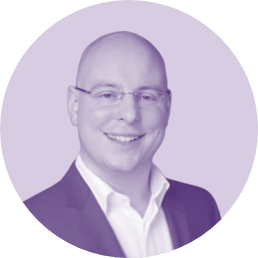
Martin Moeller
Digital Transformation Principal for Banking & Finance, Microsoft
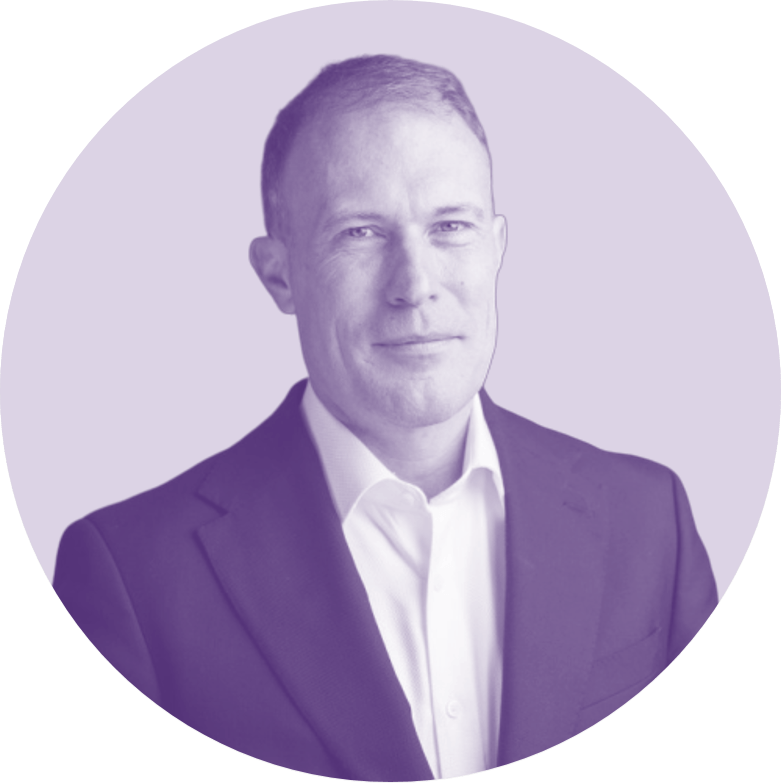
Dorian Selz
Founder & CEO, Squirro
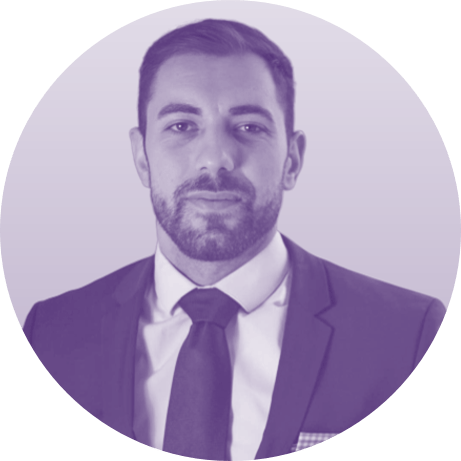
Zani Sharifi
Deputy Head, RFS
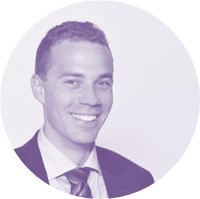
Henrique Saeuberli
Executive Briefing Consultant, IBM Research THINKLab
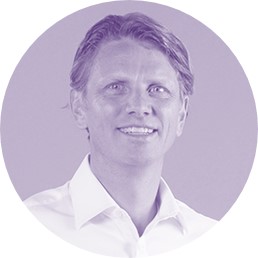
Dr. Anders Bally
Founder & Chairman, Sentifi
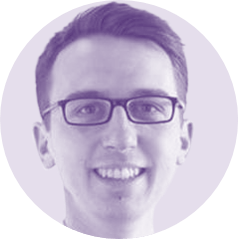
David Machac
Data Scientist, Schroder Adveq
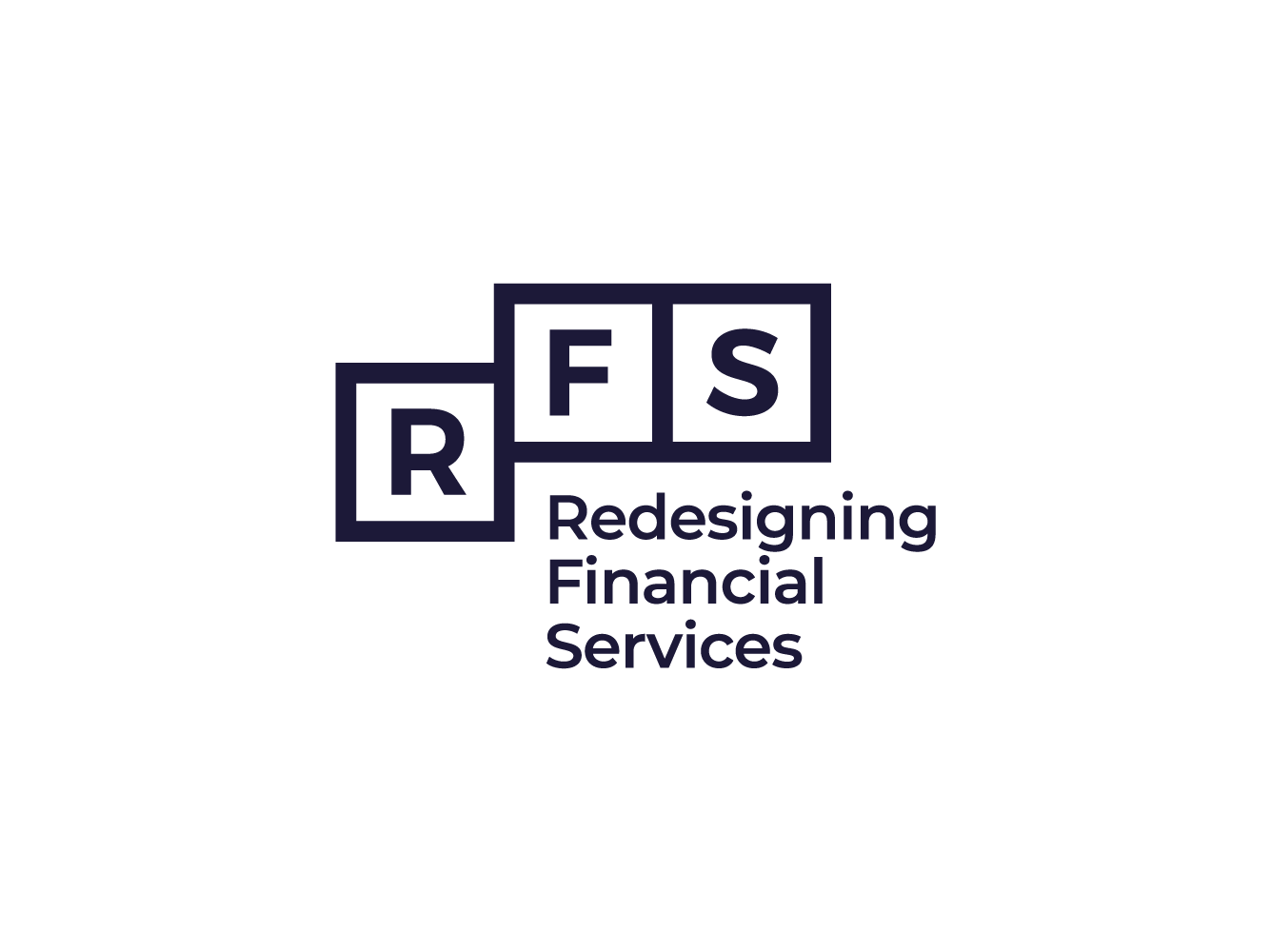
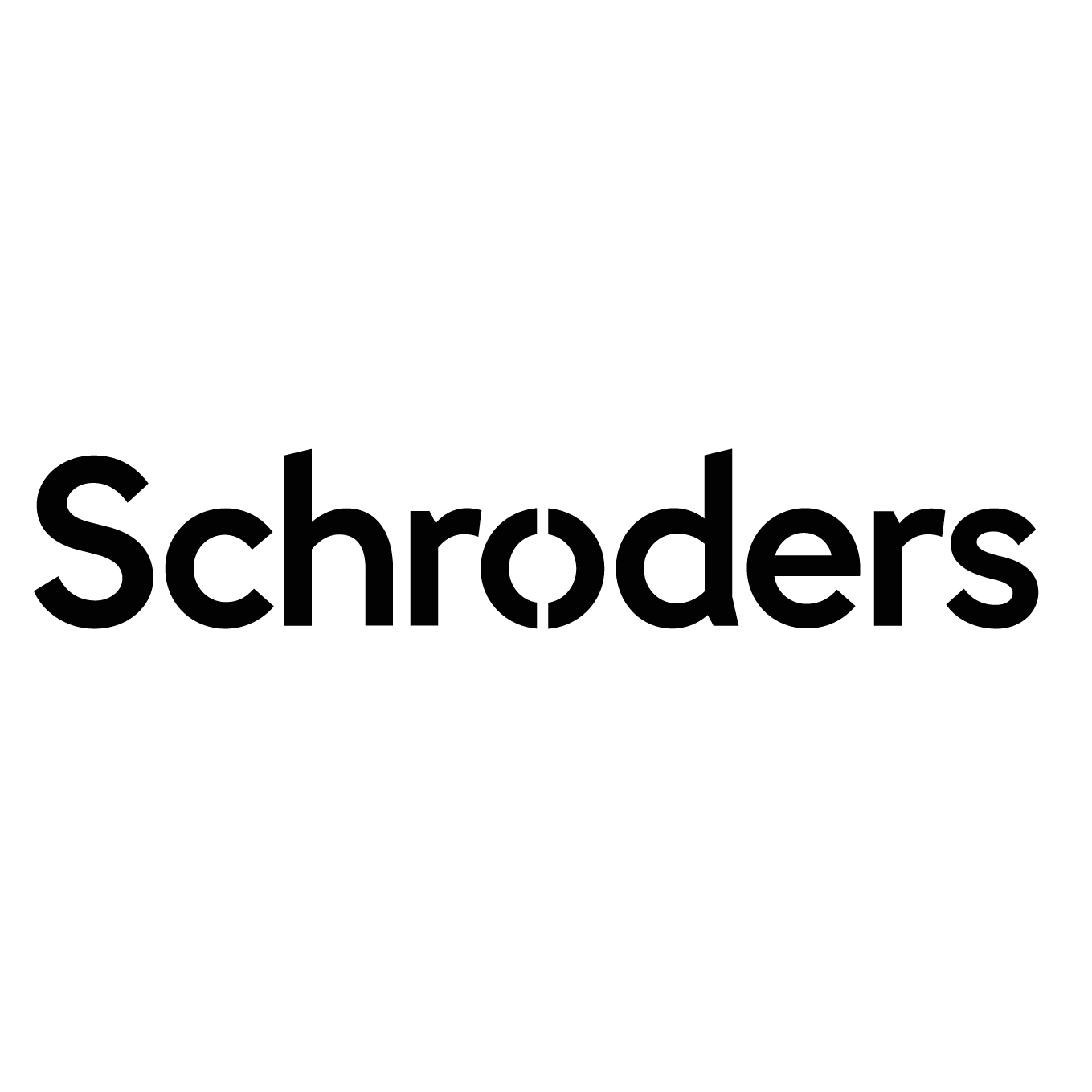